What is statistical significance
Statistical significance is determined through hypothesis testing, a method used to assess the plausibility of a hypothesis by comparing it against a null hypothesis. The null hypothesis typically states that there is no effect or difference, and the goal is to determine whether the observed data provide sufficient evidence to reject this hypothesis in favor of an alternative hypothesis.
A result is deemed statistically significant if the probability of obtaining the observed data, assuming the null hypothesis is true, is very low. This probability is quantified using a p-value. A p-value of 0.05 or less is commonly used as a threshold for statistical significance, indicating that there is less than a 5% probability that the observed results are due to chance.
Key Components of Statistical Significance
- P-Value: The p-value measures the probability of obtaining results at least as extreme as the observed ones, under the assumption that the null hypothesis is true. A lower p-value suggests stronger evidence against the null hypothesis. The choice of a p-value threshold can vary depending on the context and field of study; however, 0.05 is a widely accepted standard across many disciplines.
- Significance Level (Alpha): This is the threshold set by the researcher before conducting a test. Commonly set at 0.05 (5%), it represents the probability of rejecting the null hypothesis when it is actually true (Type I error). Researchers choose an alpha level based on the acceptable risk of making a Type I error, which could have implications for policy decisions or scientific conclusions.
- Confidence Level: Complementary to the significance level, it indicates the degree of certainty in the results. A 95% confidence level is typical, implying that if the experiment were repeated multiple times, the results would be consistent with the observed effect 95% of the time. This confidence level reflects how often the true parameter value would fall within the confidence interval if the same population were sampled repeatedly.
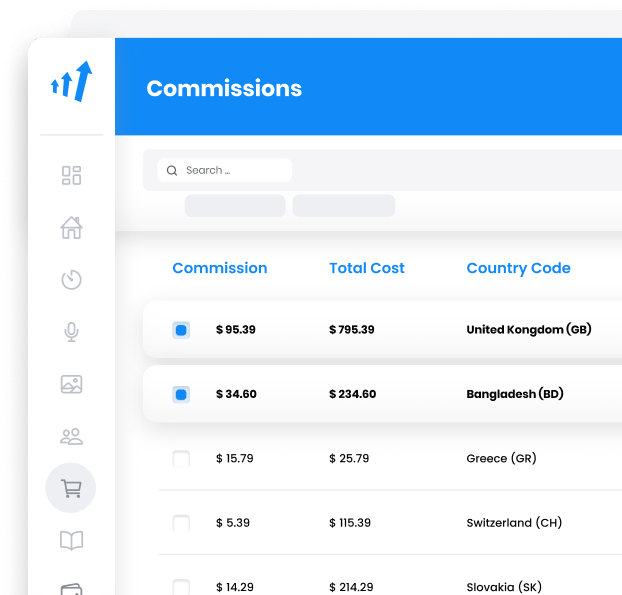
Statistical Significance in Affiliate Marketing
In affiliate marketing, statistical significance is crucial for evaluating the performance of marketing campaigns, optimizing conversion rates, and making data-driven decisions. For instance, when A/B testing different landing pages or ad copies, marketers rely on statistical significance to determine which version performs better in driving conversions. This process helps in allocating resources efficiently and improving the return on investment.
Challenges in Achieving Statistical Significance
- Sample Size: Small sample sizes can lead to unreliable results, as they may not adequately represent the population. Larger samples typically yield more reliable results. However, larger samples may also require more resources and time to collect, making it crucial to balance sample size with practical constraints.
- Effect Size: This measures the magnitude of the difference between groups. A small effect size may require a larger sample to achieve statistical significance. Understanding the effect size is essential for interpreting the practical implications of the results, as it provides context about the importance of the findings.
- Multiple Comparisons: Conducting multiple tests increases the risk of Type I errors. Adjustments, such as Bonferroni correction, may be necessary to maintain the integrity of results. When multiple hypotheses are tested, the likelihood of obtaining a significant result by chance alone increases, necessitating corrections to account for this risk.
Practical vs. Statistical Significance
While statistical significance indicates that an effect is likely not due to chance, it does not necessarily imply that the effect is practically significant or meaningful in real-world scenarios. For example, a statistically significant increase in conversion rate may not translate to a substantial revenue increase if the effect size is small. Practical significance considers whether the magnitude of the effect has tangible benefits or implications for decision-making.
Enhancing Statistical Significance in Affiliate Marketing
Continuous Monitoring: Regularly review data and adjust experiments as needed to account for changes in market dynamics. Continuous monitoring allows for timely interventions and optimizations, ensuring that marketing strategies remain effective.
Design Experiments Carefully: Define clear hypotheses and choose appropriate metrics that align with business goals. Ensuring that the experimental design is robust and aligns with the objectives helps in deriving meaningful insights.
Adequate Sample Size: Ensure that the sample size is large enough to detect meaningful differences. Calculating the required sample size before conducting the study helps in achieving sufficient power to detect effects.
Random Sampling: Use random sampling techniques to minimize bias and ensure that results are representative of the broader audience. Random sampling enhances the generalizability of findings by reducing selection bias.
Use Advanced Analytical Tools: Employ statistical software and tools to accurately calculate p-values and confidence intervals. These tools can also assist in visualizing data and performing complex analyses that may not be feasible manually.
Frequently Asked Questions
Why is statistical significance important?
Statistical significance is important because it measures how likely it is that a result occurred by chance.
How is statistical significance used?
Statistical significance is used to determine whether a result is by chance or caused by some factor of interest. If statistically significant, it is unlikely to have occurred by chance.
Why is statistical significance important for business?
Statistical significance is important for businesses for many reasons such as allowing them to measure the effectiveness of their marketing campaigns, helping them understand their customer’s buying habits, and providing useful information for making decisions about pricing and other strategic decisions.
Explore Post Affiliate Pro's comprehensive Affiliate Marketing Glossary to enhance your industry knowledge with easy-to-understand definitions of essential terms. Perfect for marketers seeking to understand the fundamentals and advanced concepts, this glossary helps you master the language of affiliate marketing. Visit now to boost your expertise!
Discover the role of an affiliate in marketing—earn commissions by promoting products through banners, links, and campaigns. Learn how affiliates drive sales and explore effective marketing strategies. Join the world of affiliate marketing with Post Affiliate Pro for user-friendly tools and exceptional support.
Discover what a search term is—a key concept in marketing that involves the text users type into search engines. Learn how to optimize your business keywords to ensure your ads appear in relevant searches, and find out how to use search term reports to track popular keywords. Explore strategies for adding or blacklisting keywords to refine your ad visibility effectively.